I routinely look at posts in my subscribed sub-Reddits that showcase new JS libraries, the latest in AI, and other fun maker projects. This time around, I stumbled into Google’s Machine Learning API blog post. Google recently released a JS web friendly interface that creates AI/Tensorflow models for images, sound, and human poses. Developers can create these models with any number of recorded collections created by the UI. The webcam references the collection of images, sound or human poses to see what matches closest to the created collection. It provides a HTML file and a JSON for your generated model and gets you bootstrapped to start your project.
After a deep dive on the topic, a fun idea struck me. The idea started when I learned our office encouraged workers to wear ugly sweaters for our winter break breakfast. So I thought of an idea to have a camera look at peoples sweaters and see if they’re truly ugly.
The goal was to develop a model that can determine if anyone’s Christmas sweater qualifies as a REAL ugly sweater. First, I would generate a collection of images that are very deliberate ugly Christmas sweaters. The second would be a collection of plain boring sweaters. The AI would then would then determine if your sweater matches either collection by percentage. So if a sweater had very little “Christmas” design on it, it would identify closer to the boring sweater model. This would give you a low matching percentage. Having a real looking Christmas sweater would rate you as higher percentage.
After understanding the core structure of the API, it didn’t take much to come up with ideas on how to make this more interesting. With previous experience in the Particle IO platform, it gave me all sorts of ideas on how to make this teachable machine project more rewarding. Particle IO is a IoT(Internet of things) platform that allows hardware connectivity through the cloud. This allows me to connect the AI framework with any sort of physical hardware. In this case, I planned in using it to trigger the LED lights after each detection and turn on my remote Christmas tree in my bedroom after the 20th detection.
Challenges
Project like these don’t always come without snags. After reading documentation from Google’s Tensorflow site, I realized the Javascript version is still fairly new. Tensorflow was developed natively for a Python platform rather than a Javascript/Node.js platform. I shied away from the Node.js route since there was hardly any support for it. So I decided to do it inline with just Vanilla since the provided code came with everything inline anyway. I would then just need to add some POST calls to the Particle.io server using their web API to trigger my LED neo-pixels strip.
The second challenge in making all this work properly was internet connectivity for my Particle Photon. Not knowing the limitations on connecting to an enterprise secured wifi connection was definitely a surprise. The Particle Photon microcontroller is cloud dependent. It relies on listening and sending commands through its cloud server. Typically, enterprise internet servers have restrictions on specific ports and the Photon uses one of those restricted ports. I had to scramble over the course of the weekend in finding a workaround for this. I gave in to the fact that I may have to use an Arduino.
I never used the Arduino platform, but I felt it wouldn’t be an huge obstacle. From reading the origins of the Particle platform, it seems like it’s derived from Arduino. The void loop() and the void setup() were familiar in the IDE environment. It also has a similar include library method just like Particle. I ordered the Arduino in Amazon and received it in a Sunday morning.
But wait. Hold up.
Now that I have detached myself from the Particle platform, I realized I was blind-sided from the fact that I did not know hot to create communication from an HTML UI to the micro-controller. From previous Photon projects, I would rely on listening to POST commands that then triggered functions in my micro-controller. It was a struggle but eventually committed to a framework called, “Involt”. It uses CSS classes to call or listen to Arduino functions. It integrated well with my async/await callbacks.
At the time of writing this, I realized there was another framework called, “P5.js“. It uses HTML canvas for most of it methods. It seems pretty straightforward and I wish I knew about it during the time of researching. I’ll definitely try it out on another project.
The same weekend I completed a functional prototype ready to show the director of our studio the next Morning. I did not want to disappoint and I needed to make sure user engagement had a good, rewarding payoff. It would not leave an impression on the user if it just “detected” a percentage of ugliness of his or her Christmas sweater. I wanted participants committed for a long term engagement. Meaning, it needed to be designed in form of a video game or TV Game show.
Code Rundown/Tools Used
The libraries/dependencies used on this project helped bring this all together. It also helped that I could develop this in plain Vanilla or else I would have dealt with dependency hell if I used other platforms that require compilers, bundlers, transpilers, or what not. This project would be too small anyway for those frameworks.
The bridge.js and framework.js are the core to the Involt framework. The bridge.js is the core library mainly to connect the Arduino through serial/USB connection. The framework.js is the default UI that is built in. It is the initial UI to for selecting the specific USB/Bluetooth port. Pixi.js and TweenMax.js was used for the snow animations after a detection. Tf.js (tensorflow) and teachablemachine-image.min.js are the main framework JS files from Google.
Particle.js was used to validate my Particle.io user credentials to make API calls to my Photon cloud. In this case, it made a POST command after the 20th ugly sweater to light up my remote Christmas tree at my home.
<script src="core/bridge.js"></script>
<script src="core/pixi.min.js"></script>
<script src="core/TweenMax.min.js"></script>
<script src="core/framework.js"></script>
<script src="core/tf.min.js"></script>
<script src="core/teachablemachine-image.min.js"></script>
<script type="text/javascript" src="core/particle.min.js"></script>
For any developers interested in the code working behind the scenes check out my project’s GitHub.
Design
The design was centered around the 8-bit style combined with the ugly Christmas sweater knit. This art direction was a no-brainer.
Even though I had a mental visualization of how the UI would look, I just didn’t have the time or resources to create the artwork from scratch. I am familiar with design and illustration but I didn’t have the bandwidth because I needed time to develop the software and hardware. Luckily, I had a last minute resource that provided key art sufficient to cover most of the UI states. I was also lucky to find a free-ware ugly sweater font.
The Presentation
I needed to set up a camera on a cafe counter top and aim it at people’s upper torso while they order their lattes. The web cam USB cable had to extend across the cafe and connect to my laptop where I had my app running. A TV monitor mounted on a wall would be connected to my laptop to display my app interface. In addition, my Arduino would be connected to the same laptop controlling the LEDs attached around the frame of TV.
Another set up was displaying the live stream of my Christmas tree at my home. At home, I had to place a Nest cam facing my Christmas tree with the LEDs turned off. The LEDs on the tree were connected to a Photon that was connected to my home’s wifi. It’s sole purpose was to wait and listen for a command from the app running at work. After the 20th and final detection, the app dispatches the command to the remote tree.
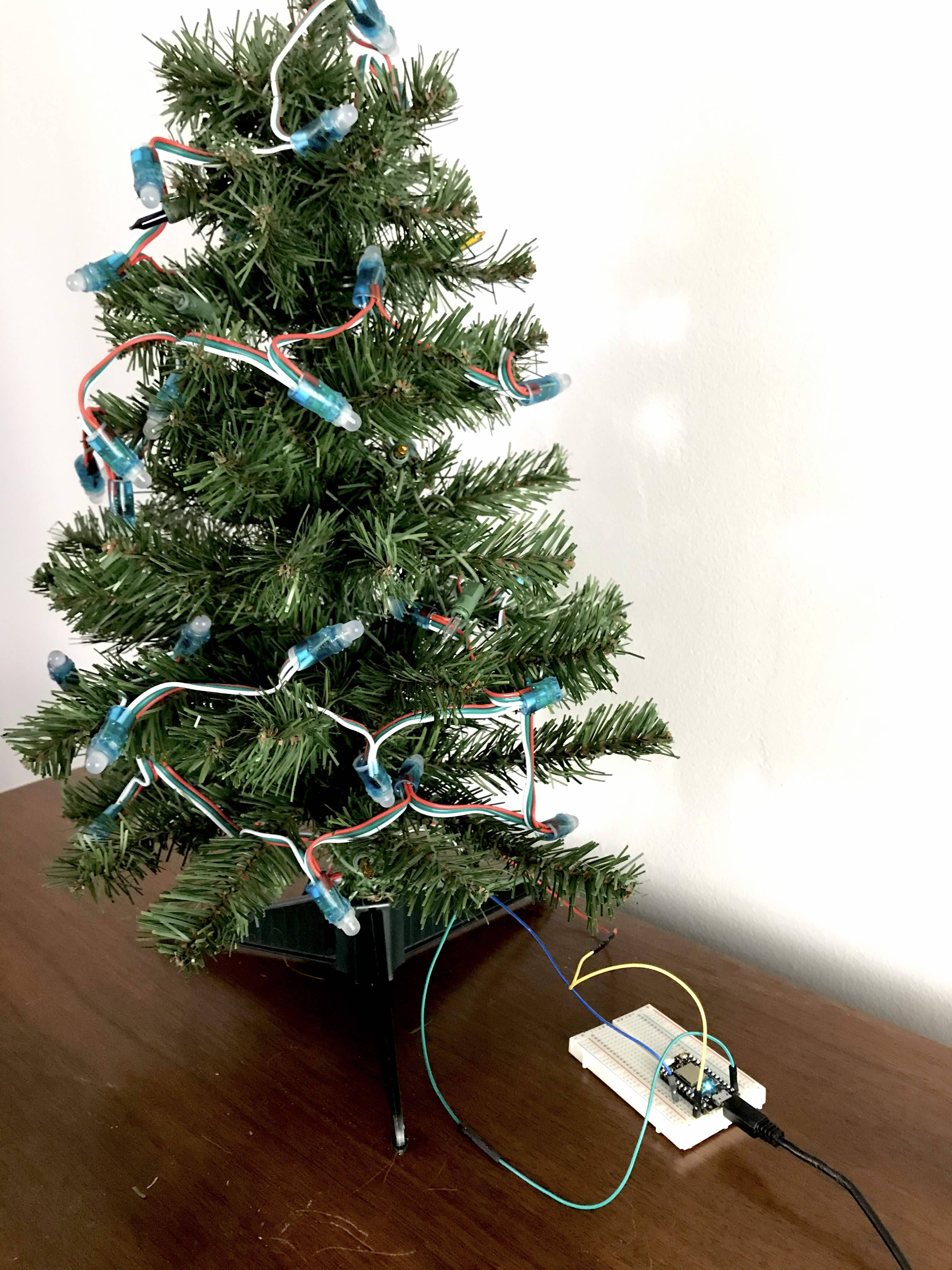
At work, I had to display the live feed at the cafe. So I needed another laptop connected to a display near the counter where people could see the tree on standby. I could have probably used the same laptop running the app, Arduino, and webcam but I didn’t want the laptop to overload and crash during the presentation.
Creating signage on the cafe counter was created to better alert people of what was going on. Just placing the camera on the counter with no signage would have been less engaging. An 11×17 inch foam board was created to call out users to show your sweater to the camera.
There was also a flyer designed the day before to announce the set up of the exhibition on the cafe. This flyer was posted on our agency’s public chat room where most workers visit every day for announcements.
Public Reaction
For the most part, people did understand the concept of the exhibit. Maybe more than half understood the instructions and the payoff. I would say 30 percent of visitors had to be guided on how to participate. The overall reaction was positive and people did have a good time getting involved as participants. It took a while to ramp up a crowd but at the end, but people were amazed of the final payoff.
Learning from the Experience
The project itself wasn’t easy to pull off. The app and hardware development was challenging but the hardest part is not knowing how the public would interact with the whole exhibit. It would have been total failure regardless of how well the software or hardware worked. Relying on every single user to understand the concept was something I knew I wasn’t going to achieve. A handful of people didn’t believe the capability of the project and thought it was all mocked. I did spent time with participants explaining how everything worked behind the scenes without being too technical.
With everything said, people were amazed and I was amazed of how well everything worked.